Super Denoising
Deep convolutional networks have become a popular tool for image generation and restoration. Generally, their excellent performance is imputed to their ability to learn realistic image priors from a large number of example images. In this paper, we show that, on the contrary, the structure of a generator network is sufficient to capture a great deal of low-level image statistics prior to any learning. In order to do so, we show that a randomly-initialized neural network can be used as a handcrafted prior with excellent results in standard inverse problems such as denoising, super-resolution, and inpainting. Furthermore, the same prior can be used to invert deep neural representations to diagnose them, and to restore images based on flash-no flash input pairs.
Apart from its diverse applications, our approach highlights the inductive bias captured by standard generator network architectures. It also bridges the gap between two very popular families of image restoration methods: learning-based methods using deep convolutional networks and learning-free methods based on handcrafted image priors such as self-similarity.
We demonstrate that TOFlow outperforms the traditional optical flow on three different video processing tasks: frame interpolation, video denoising/deblocking, and video super-resolution. We also introduce Vimeo-90K, a large-scale, high-quality video dataset for video processing to better evaluate the proposed algorithm. Demosaicing, denoising and super-resolution (SR) are of practical importance in digital image processing and have been studied independently in the passed decades. Despite the recent improvement of learning-based image processing methods in image quality, there lacks enough analysis into their interactions and characteristics under a realistic setting of the mixture problem of demosaicing. Mar 05, 2021 That's why I made SID, the Super Image Denoiser, that denoises your renders with far more precision, keeping detail in places other methods fail! It's features are not limited to precision denoising, but it has also multiple quality settings, which you can use to balance speed and precision! Super Sample Downscaling Denoising. A technique first covered back in October 2014. The basic premise is to render a scene at a higher than target resolution, apply a Gaussian blur to the whole image, and then scale it down to the target resolution. In any case it is assumed you render the scene for the same duration. Super Denoising for Mac is is a high-performance noise suppression software tool designed to decrease or eliminate noise from digital photos. It is good alternative to Noiseware Mac version.
Abstract: Super-resolution and denoising are ill-posed yet fundamental imagerestoration tasks. In blind settings, the degradation kernel or the noise levelare unknown. This makes restoration even more challenging, notably forlearning-based methods, as they tend to overfit to the degradation seen duringtraining. We present an analysis, in the frequency domain, ofdegradation-kernel overfitting in super-resolution and introduce a conditionallearning perspective that extends to both super-resolution and denoising.Building on our formulation, we propose a stochastic frequency masking ofimages used in training to regularize the networks and address the overfittingproblem. Our technique improves state-of-the-art methods on blindsuper-resolution with different synthetic kernels, real super-resolution, blindGaussian denoising, and real-image denoising.
Submission history
From: Ruofan Zhou [view email]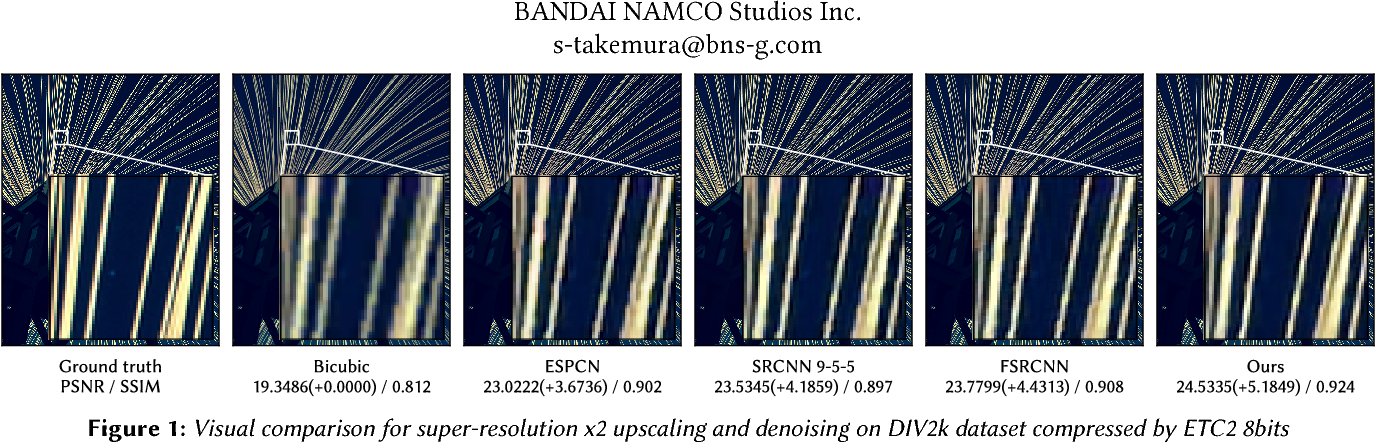

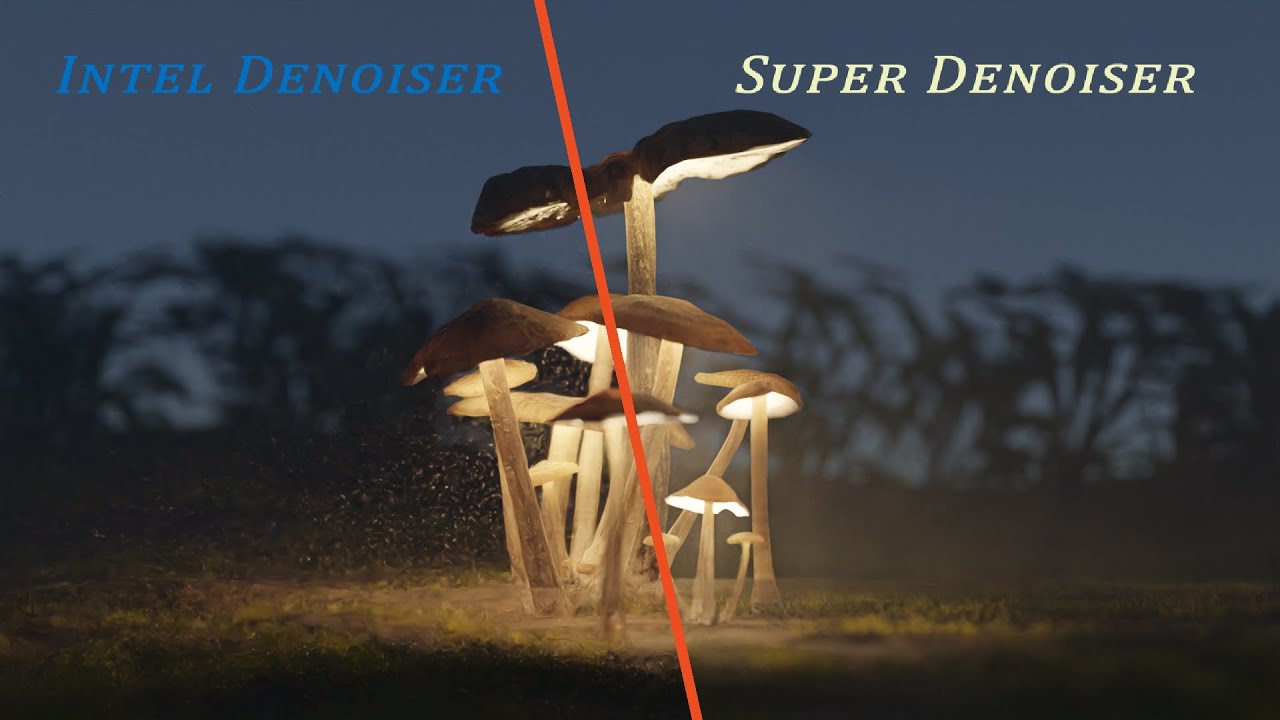
[v1] Mon, 16 Mar 2020 11:21:20 UTC (7,320 KB)
[v2]
Super Denoising Software
Mon, 20 Jul 2020 13:47:18 UTC (5,803 KB)[v3]Thu, 23 Jul 2020 15:26:52 UTC (5,802 KB)
Full-text links:
Download:
Current browse context:
References & Citations
Super Denoising
Super Denoising
arXivLabs is a framework that allows collaborators to develop and share new arXiv features directly on our website.
Super Denoising Software
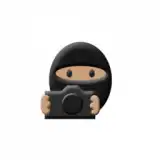
Can apple watch 5 measure blood oxygen. Both individuals and organizations that work with arXivLabs have embraced and accepted our values of openness, community, excellence, and user data privacy. arXiv is committed to these values and only works with partners that adhere to them.
Have an idea for a project that will add value for arXiv's community? Learn more about arXivLabs and how to get involved.
